Artificial Intelligence
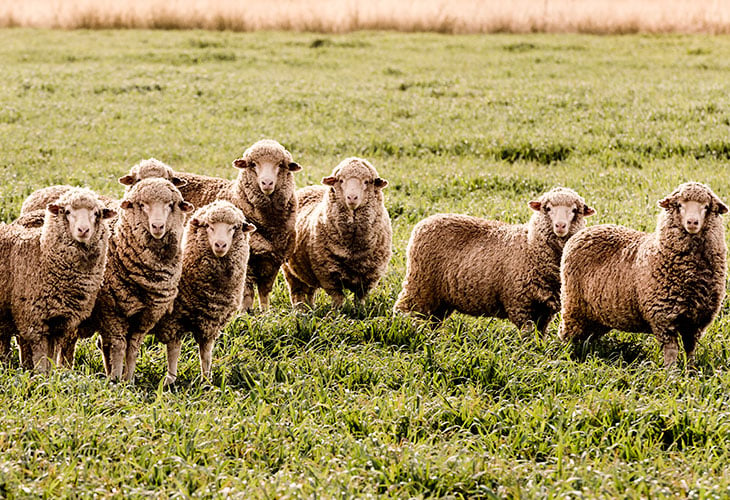
We're investigating whether Artificial Intelligence (AI) technology has potential to aid selection and productivity management decisions in a commercial wool-growing environment.
Favourable results have been achieved in an AWI-funded pilot study of whether artificial intelligence (AI) technologies can identify several key phenotypic traits in young sheep.
The project concluded that over the next five years the technology has considerable potential to aid selection and productivity management decisions in a commercial wool-growing environment.
The aim of the pilot project was to provide an evaluation of whether deep learning AI technologies could accurately predict performance indicator traits of young sheep, from analysing digital photographs of several different views of the sheep. In particular, the project examined whether AI could determine:
- Bodyweight
- Body and neck wrinkle
- Face cover
- Identify individual sheep
How AI technology works
Using both side and top cameras, the AI algorithms could predict bodyweight with an accuracy of 86% and 87% respectively. Combined information from top and side cameras resulted in an accuracy of 89%.
The device that was used on-farm by the researchers was a crate that held the sheep, and four fixed cameras – placed above, to the side, in front and to the rear of the sheep – to semi-automatically capture high resolution images and link them to sheep electronic identification (EID). The device also allowed the semi-automatic recording of bodyweight.
Using this system, the researchers created an image library of 1,482,041 images from 4,072 sheep. All sheep were weighed at the time of image capture, and subjectively scored for face cover (1-5), neck wrinkle (1-5) and body wrinkle (1-5) and identified to EID.
The AI algorithm could match anonymous face and body images with 94% and 98% accuracy respectively to sheep EID, and 99.7% when both face and body information was used. However, when images from the same sheep were tested five months later, accuracy was considerably lower (<10%) unless images from both time points were included in the training data set (accuracy increased to 90-98%), suggesting that a much larger training data set is needed with repeat images of sheep over time.
Using both side and top cameras, the AI algorithms could predict bodyweight with an accuracy of 86% and 87% respectively. Combined information from top and side cameras resulted in an accuracy of 89%.
For neck and body wrinkle the AI algorithms were able to allocate animals to either a high or low wrinkle class with 73%-90% accuracy depending which camera angle and wrinkle trait was predicted. Using the full scale of wrinkle score (1-5) prediction accuracy was lower at 38%-58%.
The project provided proof of concept that the technology works. The benefits from this project will flow in subsequent projects to this one.
While AI technology is not yet ready to be deployed in the wool industry, this pilot project clearly demonstrated that it has considerable potential. The researchers stated that they are confident that in the space of a few years they could develop a model that could outperform humans in both accuracy and speed.
As object detection algorithms improve, the researchers said could be entirely feasible to collect data in commercial settings from moving objects and make assessments instantaneously allowing for drafting and classification ‘on-the run’. In addition, movement may provide additional information on sheep health and welfare attributes.
This project led by the of University of Adelaide, has established the possibility of using OCT scanning to visualise both wool follicles and wool shafts in fresh biopsy samples. By demonstrating the feasibility of identifying both follicles and wool shafts, this technology also has the potential to provide an estimate of the number of secondary-derived follicles, where multiple shafts derive from a single follicle.
In addition, results from this project have showed that it is also possible to automate follicle density estimation using an artificial neural network algorithm.